Introduction to the bivariate copula (Bicop
) API
Import the library
[1]:
import pyvinecopulib as pv
import numpy as np
Create an independence bivariate copula
The default constructor of the Bicop
class creates an independence bivariate copula.
[2]:
pv.Bicop()
[2]:
<pyvinecopulib.Bicop>
Bivariate copula:
family = Independence
rotation = 0
var_types = c,c
Create and plot a Gaussian copula
See help(pv.BicopFamily) for the available families
[3]:
cop = pv.Bicop(family=pv.BicopFamily.gaussian, parameters=np.array([[0.5]]))
print(cop)
cop.plot() # surface plot of copula density
cop.plot(type="contour", margin_type="norm") # contour plot with normal margins
cop.plot(type="contour", margin_type="unif") # contour plot of copula density
<pyvinecopulib.Bicop>
Bivariate copula:
family = Gaussian
rotation = 0
var_types = c,c
parameters = 0.5
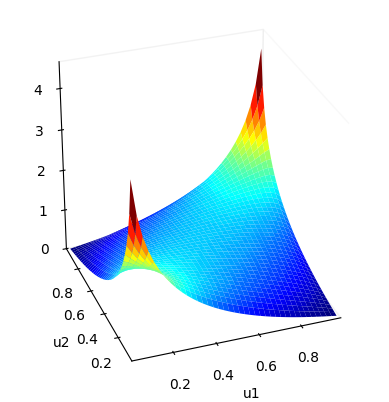
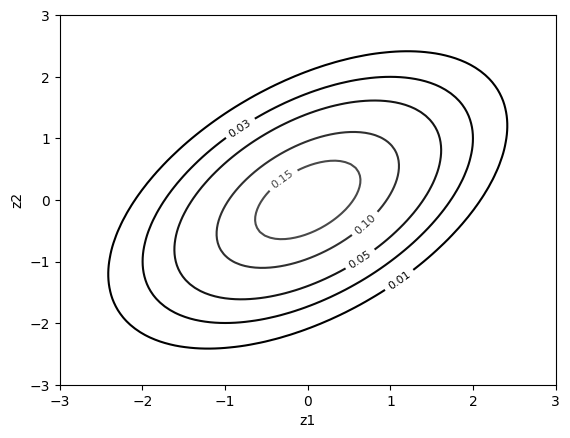
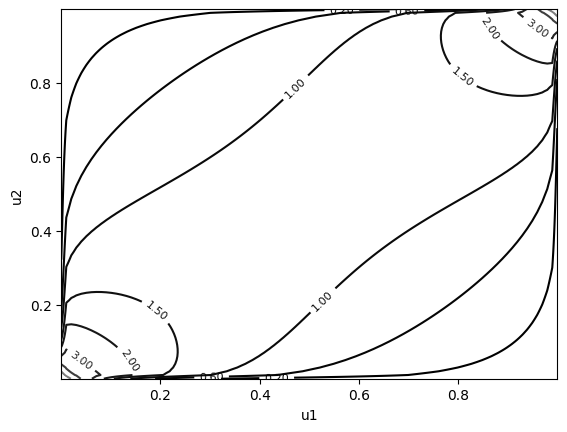
Some other families
A 90 degrees rotated Clayon copula with parameter = 3
A t copula with correlation of 0.5 and 4 degrees of freedom and showcase some methods
[4]:
print(
pv.Bicop(
family=pv.BicopFamily.clayton, rotation=90, parameters=np.array([[3.0]])
)
)
cop = pv.Bicop(family=pv.BicopFamily.student, parameters=np.array([[0.5], [4]]))
print(cop)
u = cop.simulate(n=10, seeds=[1, 2, 3])
fcts = [
cop.pdf,
cop.cdf,
cop.hfunc1,
cop.hfunc2,
cop.hinv1,
cop.hinv2,
cop.loglik,
cop.aic,
cop.bic,
]
[f(u) for f in fcts]
<pyvinecopulib.Bicop>
Bivariate copula:
family = Clayton
rotation = 90
var_types = c,c
parameters = 3
<pyvinecopulib.Bicop>
Bivariate copula:
family = Student
rotation = 0
var_types = c,c
parameters = 0.5
4
[4]:
[array([0.7092173 , 0.4810942 , 0.81301356, 1.39839611, 2.0873499 ,
2.79384106, 1.11748814, 0.55365742, 1.94610848, 0.80483478]),
array([0.13932915, 0.28749817, 0.18307038, 0.61323437, 0.0331152 ,
0.0193519 , 0.40936082, 0.18184118, 0.78248955, 0.14523531]),
array([0.8170847 , 0.11345477, 0.12286857, 0.78638305, 0.10872534,
0.35645124, 0.77472657, 0.90877192, 0.76413881, 0.77807695]),
array([0.08241333, 0.96386291, 0.79699695, 0.52499938, 0.39444021,
0.09401172, 0.33903085, 0.08152695, 0.71452328, 0.09953434]),
array([0.39910753, 0.58076221, 0.32192543, 0.81770422, 0.04078053,
0.0190836 , 0.65748713, 0.58895048, 0.92393781, 0.37153588]),
array([0.26444729, 0.79267514, 0.44179055, 0.79869798, 0.04211888,
0.01956738, 0.59671571, 0.3699726 , 0.92303602, 0.26213502]),
0.7849333316959906,
2.430133336608019,
3.0353035225961107]
Different ways to fit a copula…
[5]:
u = cop.simulate(n=1000, seeds=[1, 2, 3])
# Create a new object an sets its parameters by fitting afterwards
cop2 = pv.Bicop(pv.BicopFamily.student)
cop2.fit(data=u)
print(cop2)
# Otherwise, define first an object to control the fits:
# - pv.FitControlsBicop objects store the controls
# - here, we only restrict the parametric family
# - see help(pv.FitControlsBicop) for more details
# Then, create a copula from the data
controls = pv.FitControlsBicop(family_set=[pv.BicopFamily.student])
print(controls)
cop3 = pv.Bicop.from_data(data=u, controls=controls)
print(cop3)
<pyvinecopulib.Bicop>
Bivariate copula:
family = Student
rotation = 0
var_types = c,c
parameters = 0.52
4.1
<pyvinecopulib.FitControlsBicop>
Family set: Student
Parametric method: mle
Nonparametric method: constant
Nonparametric multiplier: 1
Weights: no
Selection criterion: bic
Preselect families: yes
mBIC prior probability: 0.9
Number of threads: 1
<pyvinecopulib.Bicop>
Bivariate copula:
family = Student
rotation = 0
var_types = c,c
parameters = 0.52
4.1
Similarly, when the family is unkown, there are two ways to also do model selection…
[6]:
# Create a new object an selects both its family and parameters afterwards
cop4 = pv.Bicop()
cop4.select(data=u)
print(cop4)
# Or create directly from data
cop5 = pv.Bicop.from_data(data=u)
print(cop5)
<pyvinecopulib.Bicop>
Bivariate copula:
family = Student
rotation = 0
var_types = c,c
parameters = 0.52
4.1
<pyvinecopulib.Bicop>
Bivariate copula:
family = Student
rotation = 0
var_types = c,c
parameters = 0.52
4.1